Jupiter Asset Management has trained its trading team on data and analytics in order to beat its rivals to the punch when finding liquidity and pricing in fixed income. Alison Hollingshead, chief operating officer (COO), and Mike Poole, head of trading, discuss the application of data science to improving internal performance and counterparty performance.
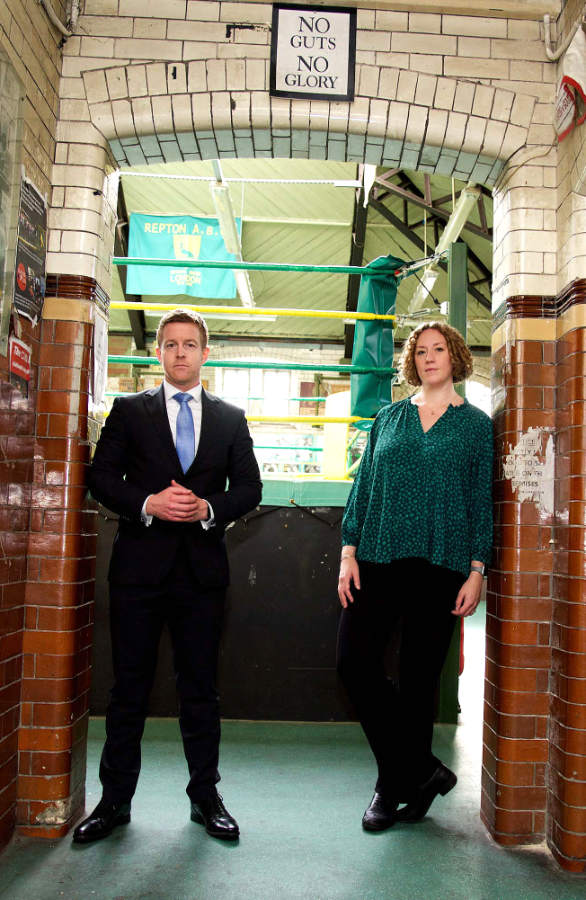
The DESK (TD): How has data science impacted broker performance analysis?
Alison Hollingshead (AH): The quality of broker reviews this year was considerably better, from a data perspective, than it has been historically. That led to so many conversations that had positive outcomes, interesting conversations with dealers acknowledging they didn’t realise they’d had some coverage change on their side, as an example.
Mike Poole (MP): It created touch points of access to the floor, suddenly we were having conversations around risk provision, we were having conversations around asset classes that those firms hadn’t known we were trading. AH: Broker reviews were historically quite informal. In this last year we have formalised the good elements which were already there, but also monitored information, getting facts and depth of data to add to those conversations. We had these really high quality reviews, a business analyst put together all these decks for us so we were employing skills that hadn’t really been there on the trading floor.
TD: What’s the most efficient way of actually improving broker performance?
MP: The best way to improve performance is by picking the right broker. You can’t do that every time, even in equities where it should be a lot easier because you have an order book, customisable algos and so you should be able to pick a counterparty to achieve what you want to achieve, but the fragmentation of the equity market and the sheer amount of volume skewed towards the close in Europe can make it v tricky. The lack of liquidity has become increasingly difficult and sometimes when looking for blocks having relationships based on conversations is key to minimising information leakage. Sometimes the first phone call you make is the most important. What we can do is use as much data as possible to make that first decision.
AH: We can then also look back with reversion analysis and tweak future decisions on that basis.
MP: Reversion analysis can be quite tricky in Fixed Income; equities is slightly easier because you can follow the price action. I would suggest some broker analysis is qualitative, more about the broker trying to understand what it is we’re trying to achieve as a business than to measure basis points of slippage versus an arbitrary benchmark. I call it ‘best execution analysis’ because you’re not looking at the same benchmarks you have in equities, like implementation shortfall and reversion. The analysis is more about making sure you used the right tool at the right time with the right counterparty without leaking information that hurting your underlying position or undermining what it is you’re trying to achieve.”
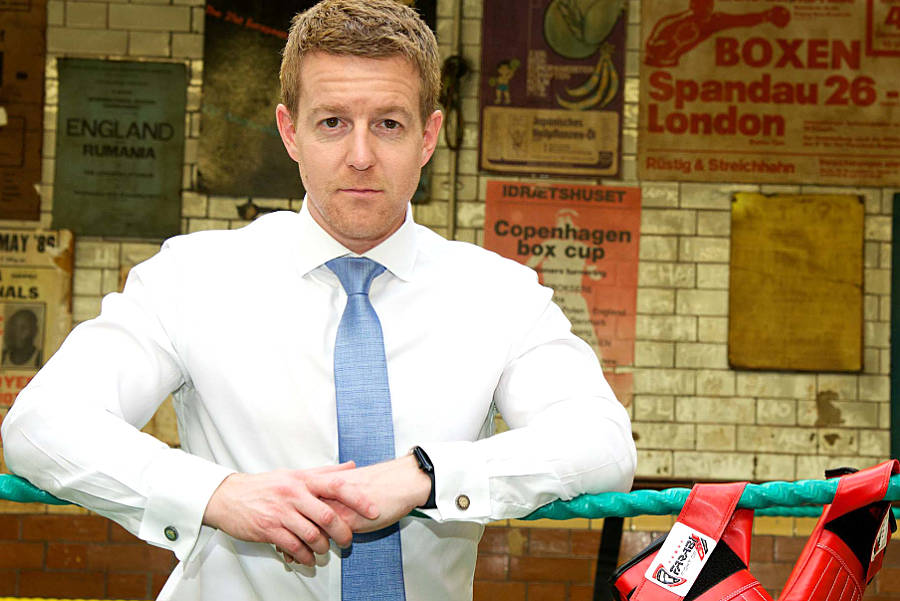
TD: What do you want from a broker ideally?
MP: If I’ve got a fund manager who is looking to add duration in a certain rating bucket, then how is a counterparty going to solve for that? Are they going to provide me risk? Are they going to show me everything they get from a portfolio trade (PT) are they going to direct me to their algo because that’s where their strength is at the moment and they’re willing to move the dial on pricing a little bit?
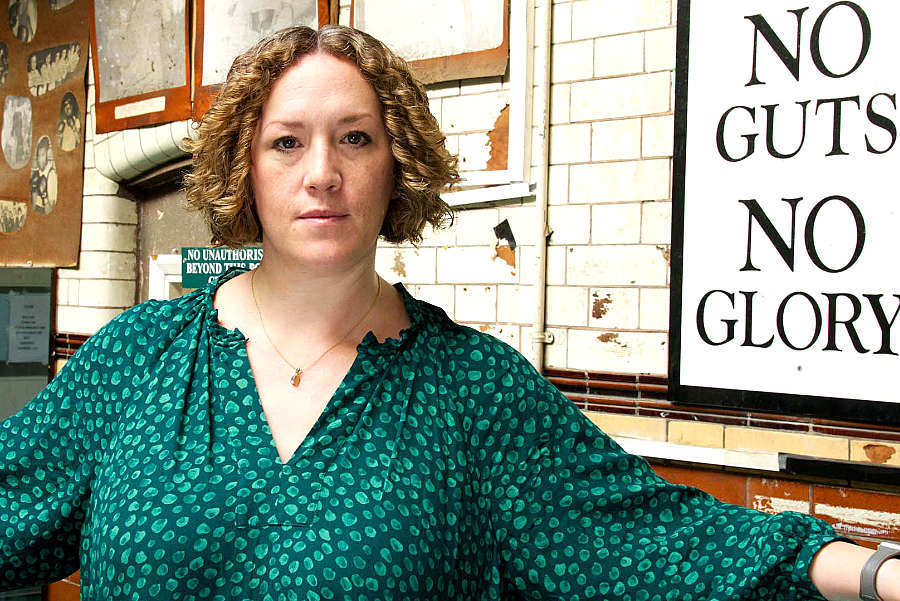
TD: Is data science built into the trading team or is it a separate team?
AH: One of the data science team almost exclusively works for the trading team now, and allows them to manipulate data, to visualise what they are trying to understand more clearly, and I have moved the data science team next to the trading desk. They can hear each other, they now sit in the centre of the investment floor. It has been really valuable.
TD: How is your team structured in terms of roles?
MP: We’ve got a very experienced trading desk with nine traders. Three equity traders have been at Jupiter for 25 years plus, I’ve been at the firm 18 years, I’ve got a fixed income trader with nearly nine years’ experience and an FX trader with nearly 15 years’ experience. The importance of having your skill set, your relationships and your ability to know how to execute well defined is more key than ever. That might sound counter to trends like automation, which we’re a big fan of in the right place. However, change for change’s sake, doesn’t always equal progress. It runs a genuine risk of change producing negative outcomes.
TD: How do you split trading expertise within the team?
MP: We have a new FX trader who is young and ambitious, and we’re now moving him into a hybrid role to learn fixed income as well. That means he can back-fill the gap left by me, as I’m not trading much anymore, so we can maintain performance without having to add headcount. I feel very strongly that when you have someone who has shown aptitude and ambition, someone who already works well within the team, and you have the opportunity to give them career progression and give them a seat on the desk, even if they don’t have a lot of experience, that is exactly what we need to do, to fill the talent gap in the industry. I struggle to see the effectiveness of bifurcation of high touch and low touch trading on a desk, because low touch becomes high touch pretty much instantaneously if there is a macro event.
TD: What is your view on multi-asset trading?
MP: I’m a fan of having traders with an experience bias. I’ve got fixed income traders with backgrounds in rates, credit, or EM and that expertise is a touch point for fund managers, sales or risk to come and talk with them about the nuances of that asset class. My EM trader can execute 100k DV01 and my gilts specialist can pick up the phone and trade futures blocks, my rates trader knows exactly where to go in high yield. That isn’t multi asset trading per se. That is fixed income trading in a smart way. An equity trader who grew up in the small- and mid-cap space will know exactly how to speak with mid-cap counterparties but they’re just as comfortable trading China onshore. I want to empower them to make the best execution decision, based on all of that expertise, rather than bucket them into what we deem to be a low-touch or high-touch order, because I don’t think that’s an easy thing to do, especially in the current macro environment.
TD: Which technologies are you using around the data?
AH: We use Aladdin which is super powerful, so we ask, how do we get the most out of the platform? How do we build around it? Are there things that it doesn’t do that we want it to do? Some of the data visualisation isn’t there yet, for example. It holds a lot of data but there are obviously places where we’ve got datasets that we want to integrate with Aladdin data, pulling data out and building our own visualisation tools over the top, so that we can interrogate kind of multiple data sets.
MP: What Aladdin has is connectivity into counterparties and trading platforms. It allows my fixed income traders to minimise the opportunity cost if there is an opportunity in the market, they are very likely to see it within the trade blotter. It would be great to go a step further and provide me and the team with analysis of opportunities we did miss, or opportunities that were there in the market. Too often, the fixed income market looks back at traded volume and execution as the sole barometer of liquidity. It has liquidity scores, everybody does these days, if something hasn’t traded for 15 days, it might have liquidity score of ‘2’. However, throughout those 15 days, there will have been opportunities to trade. They may be an order or bid wanted in competition (OWIC/BWIC) on the platform in smaller size, but nobody was willing to hit it at that time. Now, that to me shows that that bond is more liquid than the score might suggest.
TD: What operational changes would improve your access to liquidity?
MP: We always look for ideas to better identify opportunities in the market and retain that data. Having that information on a pre-trade basis would be incredibly compelling, and help us formulate ideas about how we access that liquidity with tagging axes. In equities, you have IOIs, and they all are labelled as to whether they are actionable or not. We don’t have that we have in fixed income. We have axes, which could be an indicative price, a client order, an algo or from a portfolio trade (PT). If that were clearly delineated, straight away a trader has optionality and can make a decision on how to engage with that liquidity.
TD: Are the changes you have made delivering results?
MP: We’re making a pretty good job of it. Some of that is down to standardising those datasets and making it easier to then look back and compare what we did at a given point. We’ve got TCA providers who helped us, we’ve also got an in-house data science team, who can take that TCA data, put it into a standardised turnover dashboard, and identify performance dispersion, allowing us to have a conversation with a given bank about their trading. They can then move up the rankings and the pricing we get is improved. Client outcomes have improved, simply because you have that data set visualised on a standardised base. Throughout this year, in real time, we have seen pricing improvement by having grown up conversations with our counterparties about what we’re trying to do.
©Markets Media Europe 2024