Asset managers are already able to leverage large language models to boost investment and trading workflows.
The DESK spoke with Yichuan Zhang, chief executive officer and Ivan Mihov, head of business development at Boltzbit, to discuss the practical deployment of large language models (LLMs) in investment and trading environments today, for firms of every expertise level.
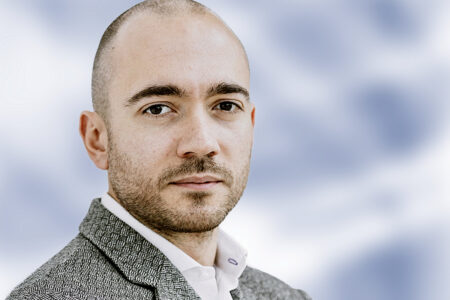
Which pain points are clients tackling through LLMs for buy-side firms?
Ivan Mihov [IM]: On the investment side, LLMs are a crucial part of the trade idea generation and portfolio construction processes, resulting in the trade orders the desk receives. This primarily involves large scale complex documentation parsing of unstructured data, information which is otherwise stuck in unusable formats, reports, emails, and delivered at too great of a volume for an analyst to single-handedly process and digest. Once data capture is solved for reliably, the information becomes a structured input into the investment thesis.
On the trading side, desks are keen to use LLMs to parse data in order to contextualise the noise, thereby improving their workflow efficiency and increasing potential alpha generation.
Traders and quants can more effectively model time-series, or non-textual data, which again needs to be normalised and labelled. The transformer technology we provide can be very useful in working with time-series and tabular data for several purposes. The first is a smarter way to select your counterparties on a pre-trade basis, a type of a recommendation and selection algorithm. The second is fair price creation – building an internal proprietary composite price, based on the firm’s data. Lastly, where a set of data is incomplete, or not sufficient, completing it by simulating synthetic data can prove useful to curve modelling and pricing it.
In essence, you can take the trade workflow from pre-trade, at-trade and post-trade stages and dissect it, in order to find two or three areas in each where an expertly trained AI agent can help. The goal is to reduce the amount of manual data work needed to reach a given point.
How expert do firms need to be to use LLMs?
Yichuan Zhang [YZ]: At a technical level the dynamic model integration with the firm needs to be managed, the technical integration with the data stream, the IT infrastructure, but also engaging with human expertise to read the data, and give feedback, to constantly improve the model. You could potentially build this up yourself, but without external capabilities it would be a year or more, and the maintenance overhead is tremendous. We have this specialised, pre-developed product to solve that issue.
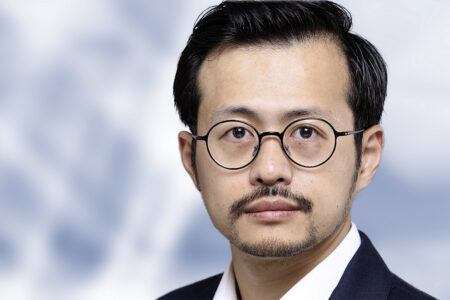
IM: There is demand at both ends of the spectrum in terms of capability. Smaller firms often lack the resources to throw at this issue, or do not have the inhouse machine learning/AI expertise to evaluate and deploy these solutions. We support those businesses with a full service offering. On the other hand, you have very large firms who have that knowledge and expertise, but want a highly customised service with high performance technology. They need customised large language models (LLM) as a Service, which we provide. They can develop the application and infrastructure with their own capabilities, and we provide the scientific intellectual property (IP). In the middle of the spectrum is a wide range of demands reflecting abilities currently unclassifiable due to the ranges of understanding. As a young company we focus on those firms and desks who are capable of moving forward at speed, be it a more tech inclined traditional investment manager, hedge funds, or even fintech companies including order and execution management system (O/EMS) providers, to supercharge their AI-driven domain specific solutions.
What specifically do publicly available language models lack that requires support?
YZ: Trading and investment workflows are not uniform, and so they need customised solutions and deployment. The product wrapper interface we offer accommodates use cases at every level. We have model components for particular use cases, like email parsing for example. Then our model architecture has been developed to be highly efficient and scalable, in comparison with off-the-shelf models. We support a service infrastructure to ensure the model being used is the right one.
IM: In some cases the model really becomes a small language model (SLM) due to the specialisation that is needed for tasks, and the user needs to be able to take that over a much larger less efficient alternative. Compute resources matter too.
YZ: Data ownership and security are key. There are very tight, secure ways of managing data, and sharing with closed generalistic models is prohibitive. Having third party IP, but also control over the system is invaluable for all financial firms.
TD: A major concern is the capacity of an LLM to hallucinate; how do you mitigate that?
YZ: Hallucination can be caused by a model not having access to the right information. This can be resolved by putting primary, proprietary document information as the context into the model. That does not solve the whole problem, so we allow our LLM to build up a chain-of-thought, transparently, to share between experts. This approach allows you to see how the AI thinks about the problem, and give direct feedback, in order to customise the AI to think in the way you think, because every firm is different and highly specialised in what and how they do it.
©Markets Media Europe 2024