SOLVE, provider of pre-trade quotes data across fixed income markets, has rolled out an artificial intelligence platform (AI) that is designed to predict the next trade level for fixed income securities. Eugene Grinberg, founder and CEO, SOLVE spoke exclusively to The DESK about how the platform works, what asset classes are next, and just how accurate SOLVE’s new AI platform is.
Quotes on bonds provided by SOLVE Px (SOLVE Price) will be generated by SOLVE’s AI model, which is designed to predict a security’s next trade price based on several hundred feature inputs, including reference data, trade data and SOLVE’s proprietary muni quote data collected from market contributors. The AI tracks 900,000 muni bonds and by having a consistent pricing time series across almost the entire muni asset class, including pricing for extremely illiquid bonds, it is hoped SOLVE clients will be able to extract broader market insights to help identify opportunities.
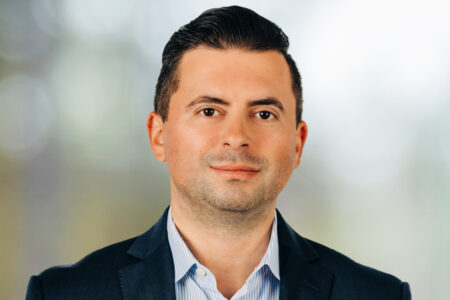
SOLVE founder and CEO Eugene Grinberg said, “The 900,000 muni bonds our AI is tracking is many multiples of the universe that sees quotes or trades.”
Talking to the DESK, Grinberg said that, in the past, a traditional linear model construction – a ‘black box’ – would have meant that there was no visibility into what some of the underlying assumptions and the simplification of a model were.
“You couldn’t see how those factors were impacting the model performance under different scenarios. Our clients would have been apprehensive of such a product,” Grinberg said. “AI is different. SOLVE Px is inherently capable of modelling complex interdependencies with very high dimensionality and non-linear relationships of the underlying features, while also retaining flexibility to continue to learn and perform under various scenarios.”
“Our model is trained on over 300 feature inputs extracted from trades, quotes, and T&C datasets. It’s making decisions on a different scale,” Grinberg added.
The new SOLVE Px platform will add a new layer of price transparency, the future-trade layer, to the decision-making process for SOLVE clients already using SOLVE’s Quotes Platform for price transparency data on more than 20,000,000 securities across different asset classes.
While SOLVE Px will initially start with municipal bonds, the platform is set to be extended to other asset classes later this year.
The SOLVE Px model generates both a bid and offer level data, depending on whether the market maker is buying or selling and will provide users with a wealth of insight that can be taken from the data, including: price, spread and yield-over-time for individual bonds; individual bond stats, including changes day-over-day, week-over-week; comparisons between individual bonds and their comparable bonds and peers; historical trends vs current performance; and comparing cohorts of bonds.
On what asset classes the AI will be working with next, Grinberg told The DESK that SOLVE has been focused on price transparency through digitisation of quotes across many asset classes in fixed income markets. “As a result, in addition to munis, we have highly differentiated data across securitised products, corporates, syndicated bank loans, converts, credit default swaps, and others.”
“Our goal is to apply AI techniques to extract larger predictive insights across all of these asset classes. We expect to announce the next asset class in the coming months,” Grinberg said.
On just how accurate the platform is, Grinberg explained that the model’s construction is to predict the next trade and to minimise the prediction error to the trade data – a task that requires continual learning and tweaks to the platform, as well as an understanding that even humans are never 100% accurate at predicting.
“It continues to learn and optimise itself with that goal. Our view is that that is the best definition of ‘accuracy’ since the fair value of any asset definitionally is what someone is willing to pay for it.”
“It also relies on the quotes data since that’s another key gauge of fair value. We continue to experiment with different methodologies and formulations of the model to drive down the prediction error.
“I don’t think any model can claim to be 100% accurate because at the end of the day many trades made by humans who interpret fair value and opportunities differently and some trades will happen off-market for various reasons – some don’t operate with the best data, others are looking to clean up the book or are willing to give up a better price for the sake of a larger relationship or better allocation of new issue,” Grinberg said.
© Markets Media Europe 2023
©Markets Media Europe 2025