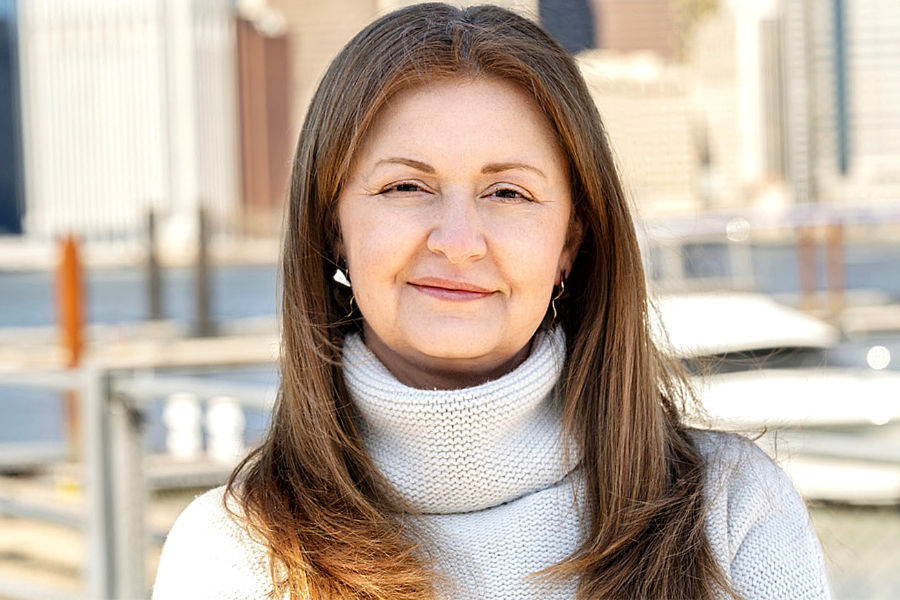
7 Chord promises to change the way AI pricing reacts and adapts to sudden geopolitical events and their aftermath.
The DESK spoke with Kristina Fan, CEO of 7 Chord, to discuss how BondDroid, the firm’s real-time AI dynamic pricing platform, adapts to sudden geopolitical events and their aftershocks and how this can impact investment and trading decisions in fixed income.
How has demand for pricing data changed this year?
Pricing is a counter-cyclical business. When the market is stable and orderly, market participants are less differentiating with respect to the quality of pricing they see from their providers. But, when the entire asset class suffers record-breaking outflows and passive investors are willing to sell at any price, the accuracy of your pricing matters a lot. It makes a difference between a profit or a loss, how much extra collateral you have to post against your financed position, or if you are hitting a stop loss.
In times of volatility, traders, investors, collateralized lenders, back and middle office professionals, and fintechs who service them need real-time pricing that accurately reflects a liquidation value of their position or indicates an attractive entry point.
We saw a similar focus on price transparency in 2020. However, at that time, the radical personal and professional lifestyle changes overshadowed many other issues. In 2022, there has been a widespread realization that we’ll be facing stagflation, volatility, and geopolitical “unknown unknowns” for a long time. Hence, a desire to upgrade the real-time price transparency tools and data feeds by stakeholders across the front, middle and back offices.
What makes your services stand apart?
The key differentiator is that our real-time pricing is fully automated and systematic. In 2022 the advantages of this approach versus using human evaluators are evident to most. However, even among automated pricing systems BondDroid approach stands apart in the current environment.
As you may know, BondDroid AI was designed to handle rapid and radical changes in the market. Most machine learning systems in production today learn the underlying distribution of the training data and apply these learnings to predict an outcome based on unseen input data. Data scientists often measure their success on how well their model generalizes on unseen data. The critical assumption is that the training and unseen data come from the same distribution. This assumption is often incorrect in practice for many reasons. The real world isn’t stationary, and data distribution may shift as market regimes change.
We live in a world where change is constant and rapid due to greater human interconnectivity, advances in technology, and automation. Successful machine intelligence systems should be built based on the assumption that data always drifts. Thus, the predictor’s essential performance requirement is its ability to adapt to change. That’s precisely where we succeeded with our approach and what we continue to perfect.
2020 schooled the market on data shifts, but 2022 brought another test of unprecedented geopolitical risk. Russia’s invasion of Ukraine has shaken the world order. This affected Ukrainian sovereign debt prices and made Russian equities and bonds uninvestable due to economic sanctions.
Immediately following the invasion, the secondary order effects were less obvious. They were harder to predict and incorporate into pricing. For example, the closure of Ukraine’s ports on the Black Sea sent prices of essential commodities soaring. Fears about the impact on Europe’s economies pushed the euro and the British pound sharply lower against the U.S. dollar.
How do you incorporate geopolitical risk into bond pricing?
Our approach to pricing is often referred to as nowcasting or microprediction. We make short-term forecasts for the near future. In particular, we predict the next bid or ask price at which a bond would be bought or sold in a competitive market. We aim to incorporate as much information available to the market at a given time. Given the far-reaching consequences of the Russia-Ukraine war, even that was a difficult task.
This is where our partnership with Tellimer will be beneficial. The Desk wrote about its first phase here: https://www.fi-desk.com/tellimer-launches-end-of-month-emerging-market-bond-pricing-data/
This collaboration is just the beginning of our work with Tellimer to improve the quality of pricing available to buyers and sellers of emerging markets’ sovereign and corporate debt. We have planned several other initiatives that will leverage Tellimer’s deep country risk expertise, advance the predictive quality of our pricing and benefit all market participants as a result.
How will research and applied pricing be provided together by 7 Chord and Tellimer?
Tellimer’s research teams sit in multiple locations around the globe and are composed of analysts with their finger on the local markets’ pulse. They have a portal where one can access their research and data. So you have primarily voice traded markets, creating pressure around price transparency and technical pressure around understanding the underlying risk. You certainly need more than just price quotes to provide accurate pricing.
We initially partnered with Tellimer in early 2021. We now provide prices through their Data Channel (https://tellimer.com/channels/tellimer-data) alongside Tellimer’s macroeconomic and country risk data.
Our next project will leverage their recently released tool called Parsel. It’s a very efficient text parsing editor, created and used by Tellimer’s research department for all kinds of documents, including International Monetary Fund (IMF) meetings and central bank reports from different countries. We plan to use it to parse Tellimer’s research reports, turn them into a data feed, and extract country risk signals to get ahead of the market. We will start with quantitative macroeconomic signals and then assess the complexity of extracting other potential signals that are more subtle. In some of these regions, where there are no principal quotes, that is potentially enormously valuable.
Which type of users will find this most advantageous?
In the past year, we’ve seen a lot of interest from collateralized lending businesses, real-time risk platforms, both 3rd party and internal, and of course, traders and investors. Potential asset sellers want to know the expected price at which they can sell as asset or to forecast their cash flow and P&L. Opportunistic and risk sensitive traders and investors, including hedge funds, can use our pricing feed to pick the bottom of the market or when to re-establish their position.
What sort of performance improvements might there be upon investing or trading?
Maintaining tradeable pricing accuracy every minute of every day requires reliable infrastructure, a lot of data aggregation, and analysis of multiple streaming input data sources. With our pricing service, traders and investors can achieve these goals without a commitment to spend $2-3mm a year to maintain the same pricing infrastructure in-house. Instead, they can focus on managing unforeseen risks. After all, historically, events we expected to occur once in 20 years now happen frequently.
©Markets Media Europe 2022